Last updated on July 10th, 2021 at 11:16 am
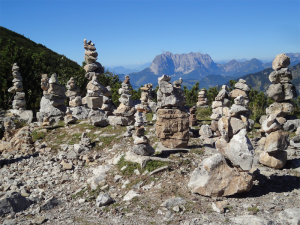
For organizations, an elegantly stated goal is one that anyone can understand, remember, and recite to others. An example for technical debt management is, “We’ll drive technical debt to zero over five years.” Or, “No project is finished if it increases technical debt.” But when the goal relates to solving a problem that has only messy solutions, stating that goal elegantly risks becoming ensnared in what I call the trap of elegantly stated goals.
Because elegant goal statements are so memorable and repeatable, elegantly stated goals spread rapidly, especially if they’re even a bit inspirational. But elegantly stated goals become traps when they incorporate overly simplistic views of how to attain those goals.
And that often happens when technical debt is involved. Here are four guidelines that can help organizations avoid the trap of elegantly stated goals for technical debt.
Beware the halo effect
The halo effect [Thorndike 1920] is a cognitive bias [Kahneman 2011]. It systematically skews our assessments of the qualities of a person, product, brand, company, or any entity, really. If our sense of one quality of the entity is positive, we’re more likely to assess as positive other qualities of that entity. The elegance of a goal statement can cause us to regard the goal as more desirable than we would if the goal were stated less elegantly. For example, the statement, “We’ll achieve zero technical debt in five years,” can increase the chances that we’ll believe that such a goal is attainable. Indeed, some might not even question its desirability, let alone its attainability.
When devising goals for technical debt management, beware the halo effect. Always question desirability, taking costs and benefits into account.
Technical debt matters less than its metaphorical interest charges
The metaphorical interest charges (MICs) on technical debt, rather than the metaphorical principal (MPrin) of the debt itself, are what matter. A goal for total technical debt might be more elegant and more simply stated than would be a goal for technical debts that carry high MICs. But goals for total technical debt can lead to effort spent on debts with low MICs. And those efforts produce little benefit.
When setting goals for technical debt management, pay attention to the MICs. Distinguish between low-MICs and high-MICs technical debt. Keep in mind that MICs can fluctuate. One kind of technical debt can be a low priority at one point in time, and a high priority at another.
Controlling technical debt is safer than trying to drive it to zero
Blind application of an elegantly stated goal can have strikingly silly unintended consequences. Keep in mind that the policymaker’s definition of technical debt is any technological element that contributes, through its existence or through its absence, to lower productivity, or depressed velocity, or a higher probability of defects.
Consider this example of strikingly silly unintended consequences for the goal of zero technical debt. An engineer creates an innovative and superior solution to a previously solved problem. Existing assets that incorporate the old solution are instantly outmoded by the innovative solution. Those existing assets now carry technical debt. If the enterprise directive mandates zero technical debt, some engineering managers might be tempted to do the unexpected. They might inhibit the kind of creativity that leads to innovative solutions to previously solved problems. The temptation arises because introducing those new solutions creates exogenous technical debt in existing assets. Therein lies the trap of the elegantly stated goal.
Throttling efforts to find innovative solutions to previously solved problems is one example of an unintended consequence of trying to drive technical debt to zero. Controlling technical debt is probably a safer option than trying to drive it to zero. Before adopting elegantly stated goals for technical debt, it would be wise to be aware of their possible unintended consequences.
Get control of the behaviors that lead to technical debt
Technical debt management efforts typically emphasize debt retirement or engineering process improvement. While both activities are worthwhile, the root causes of technical debt often lie beyond engineering. See, for example, the thread in this blog exploring nontechnical precursors of technical debt.
For example, across-the-board budget cuts can lead to technical debt. This happens because teams suspend efforts that have already created technical artifacts. If those teams lack resources needed for retracting partially implemented capabilities, the partial implementations remain in place. See “How budget depletion leads to technical debt” for a more detailed explanation of this technical debt formation mechanism.
Budget control tactics like across-the-board cuts can be counter-effective. If they don’t attend to their technical debt implications, they can add to future expenses through the MICs on the debt they generate. They can thus create future needs for budget cuts, and that leads to a vicious cycle. To gain control of technical debt, we must alter these budget control tactics. We need to provide teams with the resources they need for retracting partial implementations. That would ensure that budget reductions don’t lead to technical debt formation.
Investments in technical debt retirement and engineering process improvement are worthwhile. But they can be futile unless we first address the nontechnical causes of technical debt. It’s like bailing out a sinking rowboat without first plugging its leaks. The stated goal, “We’ll drive technical debt to zero in five years,” might better be replaced with, “We’ll get control of the behaviors that lead to technical debt within two years.”
Last words
The template known as SMART goals provides one approach to setting goals with limited exposure to the risk of elegantly stated goals. See “Using SMART goals for technical debt reduction,” for details.
Achieving control of technical debt—rather than attaining any particular level of technical debt—is a useful goal. Either we’ll control technical debt or technical debt will control us.
References
[Kahneman 2011] Daniel Kahneman. Thinking, Fast and Slow. New York: Macmillan, 2011.
- Unrealistic optimism: the planning fallacy and the n-person prisoner’s dilemma
- Confirmation bias and technical debt
- The trap of elegantly stated goals
[Thorndike 1920] Edward L. Thorndike. “A constant error in psychological ratings,” Journal of Applied Psychology, 4:1, 25-29, 1920. doi:10.1037/h0071663
The first report of the halo effect. Thorndike found unexpected correlations between the ratings of various attributes of soldiers given by their commanding officers. Although the halo effect was thus defined only for rating personal attributes, it has since been observed in assessing the attributes of other entities, such as brands. Available: here; Retrieved: December 29, 2017
Other posts in this thread
- Retiring technical debt from irreplaceable assets
- Where is the technical debt?
- Auxiliary technical debt: Rules of engagement
- Legacy technical debt retirement decisions
- Retiring localizable technical debt
- Controlling incremental technical debt
- Automation-assisted technical debt retirement
- Outsourcing Technical Debt Retirement Projects
- Refactoring for policymakers